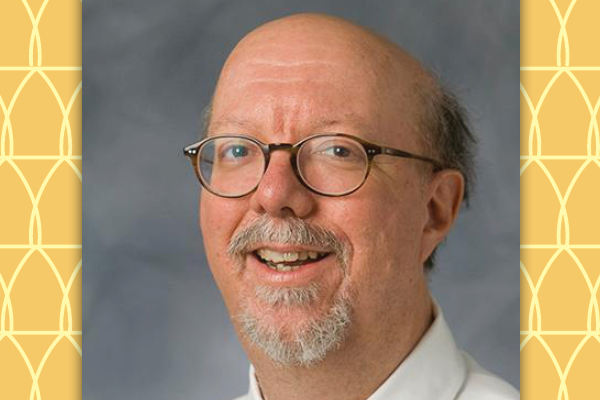
It’s all too common for cancer patients to hear their doctors tell them they are no longer responding to their current treatment. Cancer cells have a knack for figuring out ways to mutate and evolve to evade the effects of drugs. Currently, the pharmaceutical industry responds reactively — changing drug cocktails and treatment paths only after drug resistance has occurred. But what if there was a way to counter resistance before a single drop of medicine is administered?
Bruce Donald, PhD, James B. Duke Distinguished Professor of Computer Science and professor in biochemistry, and colleagues developed RESISTOR, an algorithm that uses protein structure-based computational design to predict how mutations in an enzyme will affect a drug’s efficacy. This technology could provide drug designers with insights to design better, more durable, proactive drugs.
Findings were published in Cell Systems on October 19, and the algorithm is available on OSPREY, a free, open-source software developed in the Donald lab.
Almost all cancer cells start naïve – without any resistance mutations. But like a boxer in the ring, after taking a few punches, the cancer cells evolve to learn how to bob and weave to avoid more blows.
“Tumors often become resistant to treatment over time, which leads to cancer progression, Nate Guerin, first author and graduate student in the Donald lab, said. “Being able to predict the resistance mutation ahead of time will allow for a proactive approach to treatment.”
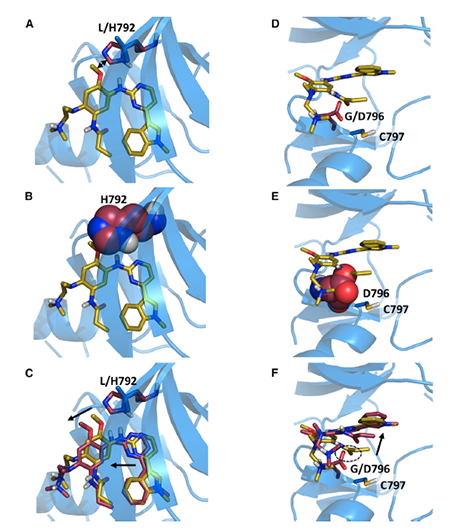
RESISTOR integrates genomics with protein structure-based and physical chemistry predictions. Collaborators at the University of Innsbruck in Austria incorporated retrospective clinical analysis and prospective studies into the algorithm to investigate how likely a mutation may occur in a given cancer type, allowing researchers to identify “mutational hot spots,” or places where mutation is most likely to cause drug resistance. Not only that, it can also predict what the mutation will be.
Using structural and genomic information, RESISTOR predicts how targets of anti-cancer drugs are likely to become resistant and what mutations they may develop. The researchers were able to look at what happened clinically, but they also tried to predict new mutations that would arise and validated those predictions by observing changes to the shape of the enzyme that are correlated with resistance.
“We searched over all possible mutations that can happen to the active site, and through detailed thermodynamic calculations, we can see which mutations could be possible,” Donald said. “By computationally solving this protein design problem, we can predict the future.”
The ability to predict resistance could help overcome one current problem: drug resistance usually doesn’t develop or become known until a drug is in active clinical use. This is true not only for cancer drugs, but also antibiotics, antivirals, and antifungals. “If you look at the packaging for Tamiflu, you find a list of possible mutations,” Donald said. “Why not fix that?”
This could help lead to a combination one-two punch. If doctors and drug developers have computational data to predict how cancer cells will respond to drug treatment, they might be able to design a treatment plan that hits cancer cells with the primary treatment and then puts them down for the count by foiling their ability to develop drug resistance.
The RESISTOR algorithm, said Donald, has the potential to help diminish one of cancer’s most powerful weapons: its ability to mutate to disable or sidestep treatments.
“Evolution is very clever, but it’s also myopic,” Donald said. “Cancer can’t predict drug design.”