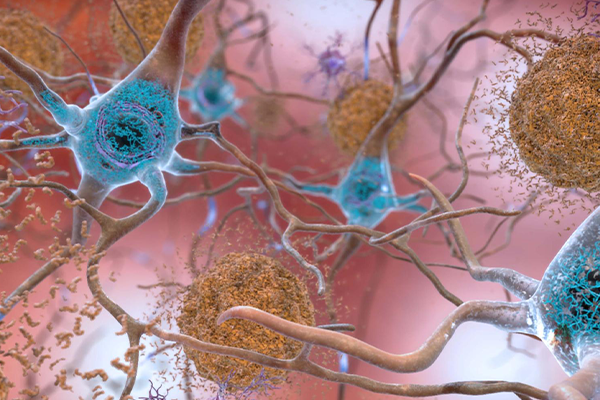
An estimated 6.2 million Americans ages 65 and older are living with Alzheimer's disease. The national Alzheimer's Association predicts that number to grow to 13.8 million by 2060, barring the development of medical breakthroughs that would prevent, slow or cure the debilitating disease.
Scientists may be one step closer to such a breakthrough thanks to a first-of-its-kind computer model that successfully simulated a clinical trial evaluating the efficacy of multiple treatments for Alzheimer's disease (AD). Jeffrey Petrella, MD, professor of radiology and director of the Alzheimer Imaging Research Laboratory at Duke University School of Medicine, was co-principal investigator, collaborating with colleagues at Penn State and the University of Tennessee, Knoxville. The study was published in the September issue of the journal PLoS Computational Biology.
“We’re calling this a virtual clinical trial, because we used real, deidentified patient data to simulate health outcomes,” said Wenrui Hao, associate professor of mathematics at Penn State, lead author and co-principal investigator. “What we found aligns almost exactly with findings in prior clinical trials, but because we were using a virtual simulation, we had the added benefit of directly comparing the efficacy of different drugs over longer trial periods.”
Using clinical and biomarker data, the researchers built a computational causal model to run virtual trials on the FDA-approved treatment aducanumab, as well as another promising therapy under evaluation, donanemab. The two drugs are some of the first treatments designed to work directly on what may cause the disease, instead of just treating the symptoms.
The researchers set the trial timeframe for both medium-term (78 weeks) and long-term (10 years) periods with low-dose (6 mg/kg) and high-dose (10 mg/kg) regimens for aducanumab, and a single-dose regimen (1400 mg) for donanemab. These are the same doses used in the human trials for FDA-approval.
Their results confirmed what was found in actual clinical trials. Both drugs had a large and sustained effect on removing beta-amyloid plaques, a peptide found in the brains of people with Alzheimer's disease. The team also found both treatments had a small effect on slowing cognitive decline in patients, though donanemab was slightly more effective than aducanumab over a simulated 10-year period.
“With over ten antiamyloid therapies in development, an important question is which one is better,” Petrella said. “It often takes tens of millions of dollars and many years to do a head-to-head comparison of drugs. Our study showed that the effect of these two anti-amyloid drugs on slowing cognitive decline is actually quite modest — and if given late in life, barely detectable.”
Petrella explained that questions remain within the medical community regarding the efficacy of removing amyloid plaques and whether or not the treatment, which is delivered intravenously on a monthly basis, actually prevents or delays cognitive decline.
“This uncertainty, combined with the 99% failure rate of trials of other classes of AD treatments, is rooted in an incomplete understanding of the complex mechanisms resulting in AD, and how disease trajectory and response to treatment may vary individual-to-individual,” the researchers write. “It is likely, therefore, that personalized treatment will need to play a central role in the future management and counseling of patients with AD.”
The researchers also used their model to develop personalized treatment plans for individual virtual patients, taking into account the potential side effects of anti-amyloid therapy such as brain swelling and bleeding, headaches, dizziness, nausea, confusion and vision problems. The team’s results show that the optimal treatment regimen gradually increases the dose until it reaches a maximum dosage and continues at a steady state.
“Our objective was to minimize cognitive decline while also minimizing the treatment dosage to limit the corresponding side effects,” said Suzanne Lenhart, Chancellor's Professor of Mathematics at the University of Tennessee, Knoxville, who collaborated on the study. “Our model will give the optimal treatment level over time of the drug, but maybe even more importantly, it provides the optimal personalized treatment plan for each patient.”
Using the framework they developed, the researchers will now look to apply optimal treatment computational modeling to other single and combination AD therapies that are currently under evaluation and incorporate new clinical trial data into their model as it becomes available.
The researchers acknowledged that such virtual trials incorporate numerous evidence-based assumptions regarding disease pathogenesis, therapeutic mechanism, side effects and a host of other factors which could impact outcome.
“Despite these limitations, this is the first step towards tailored clinical trials,” Petrella said. “We’ve shown that this type of model can work. I envision it being used as a precision tool to enhance actual clinical trials, optimizing dosages and combinations of drugs for individual patients.”
The work was supported by the National Science Foundation.