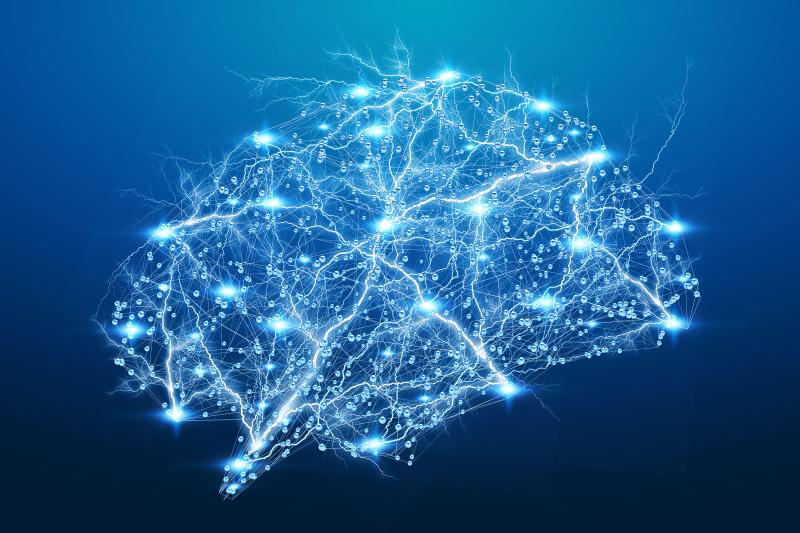
Understanding and treating brain disorders such as tremor, imbalance, and speech impairments requires deep knowledge of the cerebellum, a part of the brain that’s crucial for making accurate movements. Scientists have long been able to eavesdrop on and record the electrical signals transmitted by neurons (brain cells) in the cerebellum, allowing them to observe the signals entering and exiting this region. But the computations that the brain performs between the input and output have been largely a mystery.
Now, neuroscientists at Duke University School of Medicine, as part of a team of 23 scientists from seven institutions, have created an artificial intelligence tool that can identify the type of neuron producing electrical signals recorded from the cerebellum during behavior, allowing a new understanding of how the cerebellum works. The tool, a semi-supervised “deep learning classifier,” will allow researchers to understand the cerebellum's role across many behaviors.
The study is published in the current issue of the journal Cell.
For Stephen Lisberger, PhD, the George Barth Geller Distinguished Professor for Research in Neurobiology and one of seven co-senior authors of the study, this discovery marks a pivotal moment, promising to help answer questions he’s been pondering for more than 50 years.
“When I started graduate school in 1971, we knew that there was a circuit in the cerebellum — that neurons were interconnected in a very specific pattern. But we recorded only the input neurons and the output neurons,” he said. “We couldn’t figure out how the signals that came into the structure got transformed into the output signals. We couldn’t say how the brain did it.”
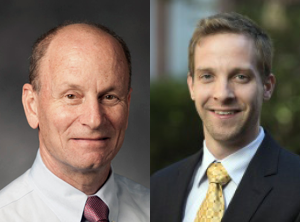
Today, neuroscientists can record from dozens of interconnected neurons at once, measuring the electrical signals generated by many neurons. In the cerebellum, like many brain areas, different neuron types are connected in consistent ways, forming “circuits” that perform the brain’s “computations,” said Lisberger, chair of the Duke Department of Neurobiology.
But the advanced techniques used to record electrical signals don’t reveal which neuron type generated them. Are these signals from Purkinje cells, molecular layer interneurons, Golgi cells, or mossy fibers? For that, neuroscientists use imaging or other types of studies. The new tool promises to combine the capabilities of both of those investigations into one.
“If you can answer how the circuit works, then you can say how the brain generates behavior,” Lisberger said.
Javier F. Medina, PhD, of the Baylor College of Medicine, the senior corresponding author of the study, compared recording the activity of neurons to overhearing a conversation between groups of people who each speak a different language, all talking at once. “Our new AI tool allows us to determine which group each recorded neuron belongs to by identifying the language it’s using, based on its electrical signature,” Medina said. “This is a revolutionary advance because it solves the first step toward decoding the content of neural conversations – understanding who is speaking. With that in place, the door is now open to uncover what the different neurons are saying to one another.”
The team from Duke, Baylor, University College London, the University of Granada in Spain, the University of Amsterdam, Bar-Ilan University in Israel, and King’s College London worked together since 2018 to create the classifier tool and validate its accuracy.
To build the classifier, the scientists first had to measure the unique electrical signatures of the different types of neurons within the cerebellum. Using optogenetic experiments, in which genes for light-sensitive proteins are introduced into specific types of neurons, the authors “tagged” the electrical activity for each cerebellar neuron type. Using these electrical signatures, they trained their deep learning classifier to sort the activity recorded from the cerebellum by neuron type.
David Herzfeld, PhD, senior research associate in the Duke Department of Neurobiology, is one of seven co-first authors of the paper. He along with colleagues from other institutions, including co-first authors Maxime Beau and Federico D’Agostino, designed and trained the classifier.
This tool is a major advance in our ability to investigate how the cerebellum processes information. — David Herzfeld, PhD
Of Herzfeld, Lisberger said, “He is a neuroscientist, he’s a machine learning guy, he’s everything.”
“This tool is a major advance in our ability to investigate how the cerebellum processes information,” Herzfeld said. “By identifying neuron types while measuring neural activity, we can ask new questions about how the cerebellum transforms inputs into outputs. I hope our techniques inspire researchers studying other brain regions to build tools that match neural activity to neuron identity, helping to uncover how different circuits function and ultimately paving the way for new approaches to treating neurological disorders.”
Other Duke authors are Marie Hemelt, PhD; Court Hull, PhD; and Nathan J. Hall.
The work was funded by the National Institutes of Health, the European Research Council, the Wellcome Trust, the European Molecular Biology Organization, the European Union’s Horizon 2020 research and innovation programme, and the SYNCH project funded by the European Commission.