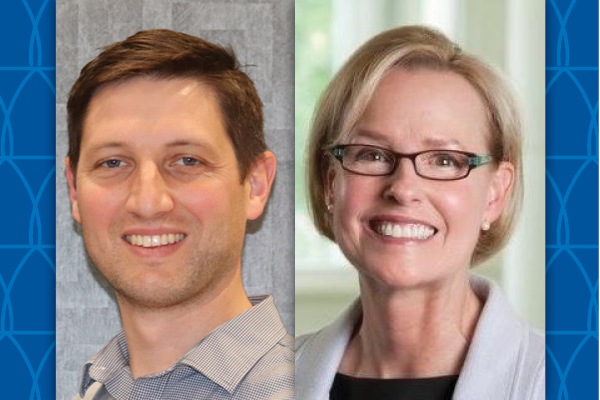
Signs of autism can hide in plain sight, but a Duke University School of Medicine study shows machine learning may help spot them.
An autism screening tool could predict likelihood of autism within one month of birth using routine medical information found in a child’s electronic health records (EHRs), according to a Duke study published Feb. 2 in JAMA Network Open.
Researchers found nearly half of autistic children could be identified at 30 days by scanning EHRs for specific patterns of health care use, including visits for neurological and gastrointestinal issues and early autism-related conditions such as problems with sleep and vision.
“We have substantial information about who is most likely to be diagnosed with autism and we have that information very early in a child’s life,” said lead study author Matthew Engelhard, MD, PhD, assistant professor in the Department of Biostatistics and Bioinformatics.
“The screening tool did a very good job of distinguishing a child’s likelihood of being diagnosed with autism later on even among children with co-occurring neurodevelopmental conditions, including attention deficit hyperactivity disorder (ADHD),” he said.
An estimated 1 in 44 children have autism spectrum disorder and health care providers often use the Checklist for Autism in Toddlers (M-CHAT) to reach the diagnosis.
The EHR-based screening tool was comparable to the M-CHAT administered for children ages 18 to 24 months, and the tool’s accuracy improved further by age 1.
The study findings suggest that combining EHR information with caregiver surveys could improve the accuracy of early autism screening, enabling families to gain more timely access to early support and services.
“Early detection of autism is important so children can begin services and therapies as soon as possible,” said senior study author Geraldine Dawson, PhD, clinical psychologist and director of the Duke Center for Autism and Brain Development. “Access to such services has been shown to improve children’s outcomes.”
Although autism can be diagnosed by 18-24 months using tools such as the M-CHAT, the median age of diagnosis is much later at 50 months, implying that most autistic children are still identified too late to fully benefit from early therapy.
For the study, researchers used EHR data from 45,080 children, including 924 who met the autism criteria.
The machine learning algorithm was developed “to detect patterns in health records that a single doctor would not be able to see unless they were spending hours churning through each individual patient’s chart,” Engelhard said.
However, the autism predictive tool can only be applied if EHR data are available for the first year of life.
Study authors say the screening tool needs further testing before it is put into providers’ hands. Researchers are exploring how to incorporate the predictive algorithm into routine clinical care at Duke Health.
The National Institute of Child Health and Development funded the study (P50HD093074), with additional support from a National Institute of Mental Health grant (ROIMH121329) awarded to Dawson.