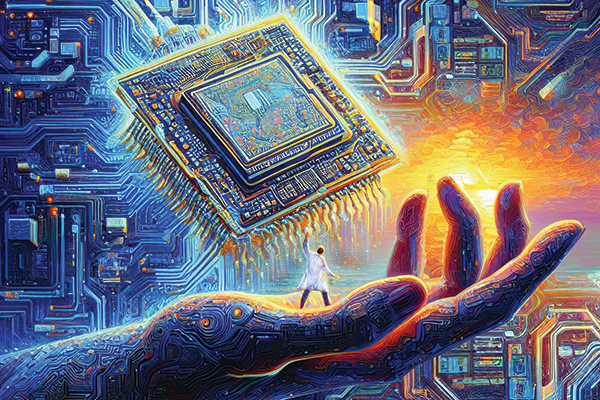
Clinicians, researchers, and educators at Duke University School of Medicine and across Duke Health are using artificial intelligence (AI) to schedule surgeries more efficiently, give students immediate feedback on academic writing, and help speed up drug discovery.
These varied applications have one thing in common; they are pointed to well-defined tasks. That’s one crucial characteristic for trustworthy AI, especially in health care, said Michael Pencina, PhD, director of Duke AI Health and, as of August 2023, chief data scientist for Duke Health.
“It’s very important that AI technology serve the humans,” Pencina said. “It’s very powerful, but it’s just math.”
Helping People, Not Replacing Them
In June 2023, researchers from Duke Health published a study in the Annals of Surgery showing that three artificial intelligence (AI) models trained on data from thousands of surgical cases were 13% more accurate in predicting operating room time needed for each procedure, compared to human schedulers alone. These AI models are now in use across all operating rooms at Duke University Health System.
The practice makes more efficient use of surgical suites and surgeon time, said Wendy Webster, MA, MBA’04, FACHE, interim assistant vice president for Duke University Health System perioperative services and director of clinical operations and health care analytics for Duke Surgery and Duke Neurosurgery. Cutting down on the number of surgeries that extend past regular working hours can reduce labor expenses for overtime. And more accurate predictions make it easier to plan a series of surgeries while using fewer operating rooms, she said.
But the algorithms don’t replace people; they help them do their jobs better.
"The human schedulers are the conductors of the orchestra."
Wendy Webster, MA, MBA’04, FACHE
An anesthesiologist “runs the board” — an electronic panel that draws from a shared calendar system built into the electronic health record. The board provides real-time progress updates for each surgeon. “They are really looking at, based on the time, how people are doing today, how the cases are progressing, where do we need to start to move people around and follow,” she said.
The predictable workflow builds trust between the scheduling team and the surgeons, and it helps increase the number of surgeries that can be performed, giving patients better access, Webster said. “The secret sauce is that we went to the end user at the beginning and asked, ‘Is this going to make their life more efficient?’ Then you’re side by side with the surgeon or the anesthesiologist or the nurse validating the data.”
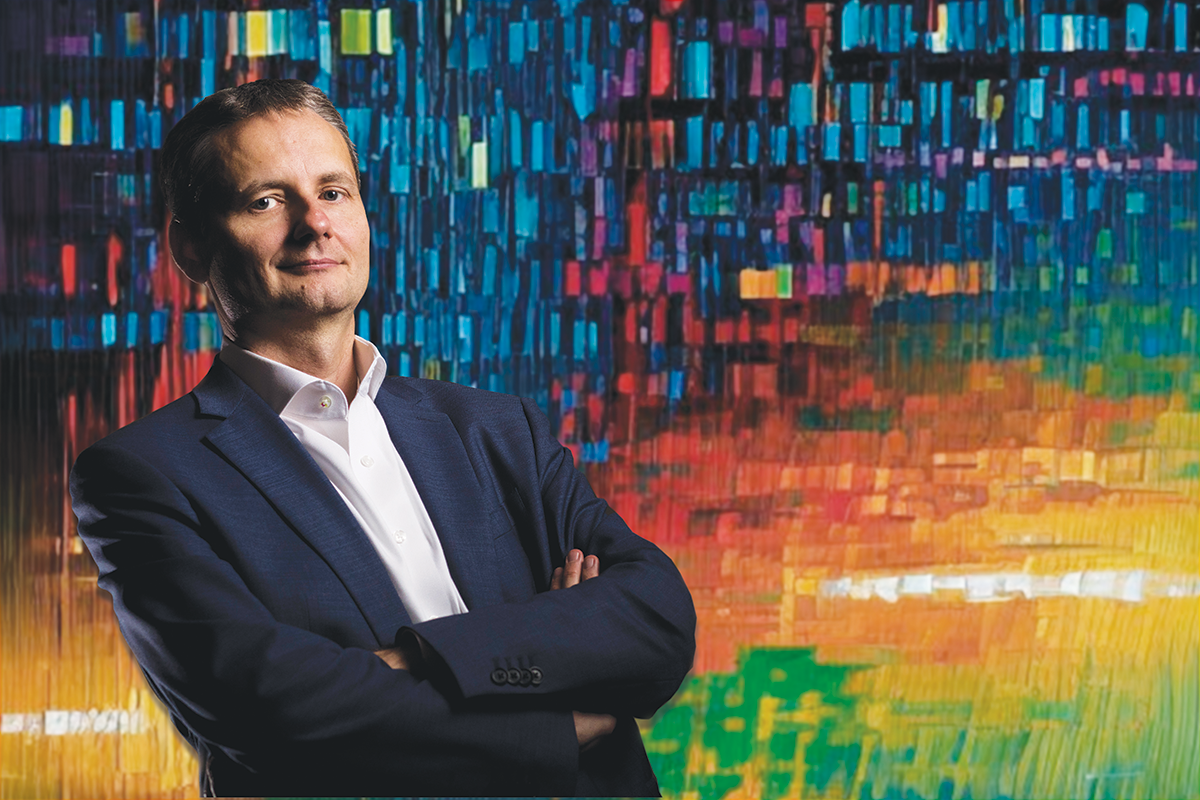
Photo by Chris Hildreth. Background generated by AI.
Another promising application of AI includes analyzing images such as mammograms to detect disease earlier. “Are there patterns in imaging that humans are missing that could be identified early on?” Pencina said. Imaging is one topic of discussion in the five-year partnership between Duke Health and Microsoft launched in August 2023 to responsibly and ethically harness the potential of generative AI and cloud technology to redefine the health care landscape.
“The conversation we’re having is circling around creating a reference standard for imaging of what healthy is,” Pencina said. “Then we can start looking at disease.”
A Boon to Drug Discovery
You’ve probably heard of or even used one of the most popular forms of AI: ChatGPT, which has exploded in popularity in the last year and is being used in classrooms in the Duke Department of Biostatistics and Bioinformatics. ChatGPT is what is known as a large language model, which can “train” itself on books and articles and “learn” the patterns and connections among words. When a user gives it prompts and asks it to create new content, ChatGPT instantly generates a written response.
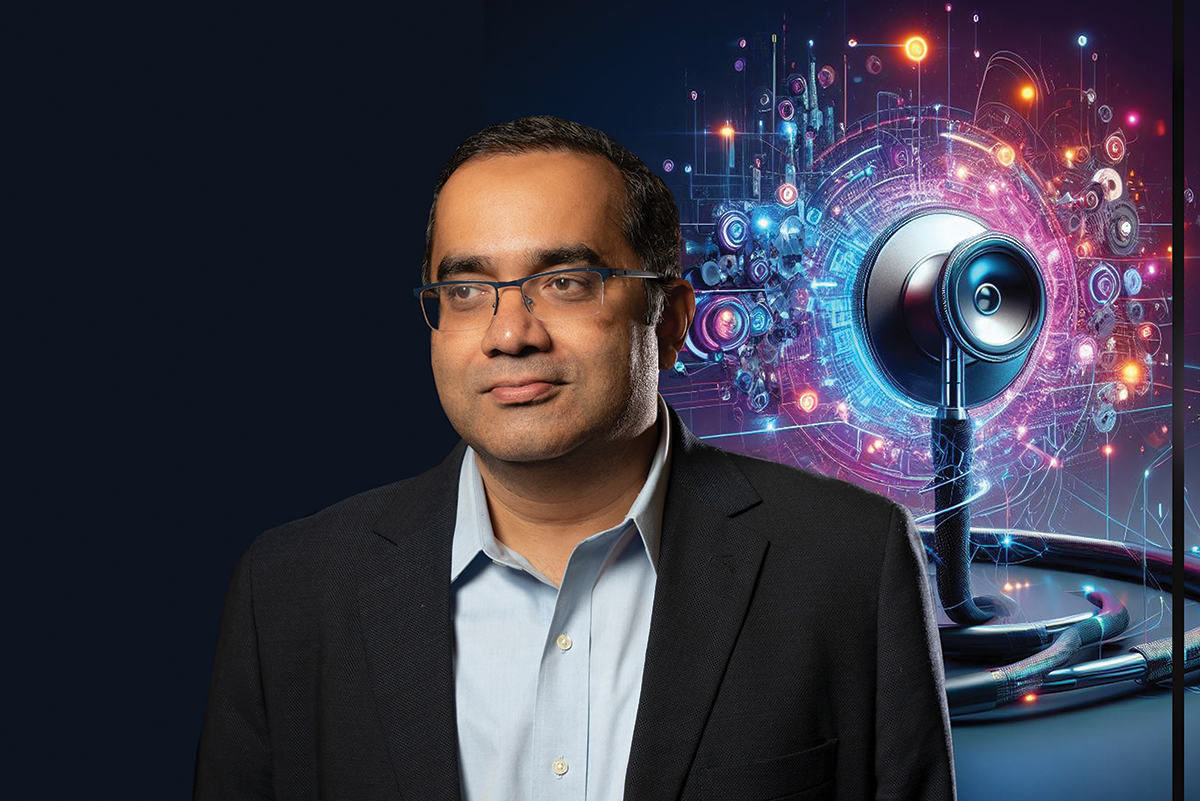
Photo by Les Todd. Background generated by AI.
Researchers at Duke are using protein language models that work on a similar principle to predict interactions among proteins and interactions between proteins and potential drugs.
"The reason it works so surprisingly well is because the way evolution has constructed proteins and the way we humans have evolved language seem to have similarities."
-Rohit Singh, PhD, a new faculty member in the Duke Department of Biostatistics and Bioinformatics and the Department of Cell Biology
Proteins are made up of chains of amino acids. The sequences of these amino acids determine the 3D structure of the protein, which governs how a protein folds and how it can bind to other proteins or to therapeutics. “We know a lot about amino acid sequences of proteins but not very much about 3D protein structure, which is what determines the protein’s function,” said Scott Soderling, PhD, chair of the Duke Department of Cell Biology.
The correlation of amino acid sequences to 3D structure has “an order and a pattern,” Singh said, not unlike human language. By training protein language models with the large amount of data available about amino acid sequences, these models can make predictions about structure that are more accurate than humans can achieve by themselves.
For many years, scientists have been determining the 3-D structure of proteins by dissolving them in a solution, crystallizing them, then using x-ray analysis, Soderling said. Using protein language models can help reduce or skip that laborious step.
Soderling said that using this and other AI approaches to find patterns in complex, large datasets will help scientists generate new hypotheses in basic and translational research that wouldn’t be possible without AI. “It will replace some things, but it also will enable us to do new things,” he said, including designing new proteins with therapeutic value that don’t exist in nature, to better target certain diseases.
Discovering and developing a successful new drug takes an average of roughly 10 years and costs over $1 billion, Singh said. “It costs you this much because you’re also folding in the cost of all the unsuccessful attempts you have made.” Drugs may be tested first in a collection of cells in a test tube, then in a mouse or other animal model, and ultimately in human clinical trials.
“But imagine if we could do all of this in the computer before we spent a bunch of money trying it out,” Singh said. “Machine learning can help us get much better at extrapolating from the simple examples to the complex examples.”
Building a Better Algorithm
In addition to designing new therapies, scientists use AI to predict disease risk. Finding out how to prevent disease is a long-standing passion for Pencina. “I lost my grandparents early on as a teenager due to cardiovascular disease, which right now we would say was definitely preventable,” he said. Before coming to Duke in 2012, he spent a decade evaluating algorithms for the Framingham Heart Study, a long-term cohort study, now in its 75th year, aimed at identifying factors that contribute to cardiovascular disease.
His research shows that caution is needed in using AI in risk prediction. In a study published in January 2023 in JAMA, his team compared algorithms designed to predict stroke risk and found that those based on machine learning didn’t perform better than standard, existing risk scores. The study also found that all the algorithms, including AI-based ones, performed worse in Black participants than white ones, although the risk of stroke is higher among Black patients.
“Disparities can potentially become propagated by these algorithms,” Pencina said. His study also found that a simple algorithm that asked patients about their risk factors, health status, and their impressions of their own health performed as well, if not better, than algorithms that used more precise measurements. “That’s telling me that patient engagement in risk prediction is very important,” he said. “We need to better understand which variables are important, which ones we need to be collecting, and how to build these algorithms in a more equitable way.”
The JAMA study is just one example demonstrating that health equity has to be “baked in” to machine learning algorithms from the start, said Michael Cary, PhD, RN, the Elizabeth C. Clipp Term Chair of Nursing and the inaugural AI Health Equity Scholar. A former practicing nurse, Cary is now a health services researcher dedicated to ensuring health care algorithms will inform clinical decision making and improve outcomes for patients. His career veered into AI when he launched a project at Duke to develop an algorithm to predict risk of decline and mortality in older adults more accurately.
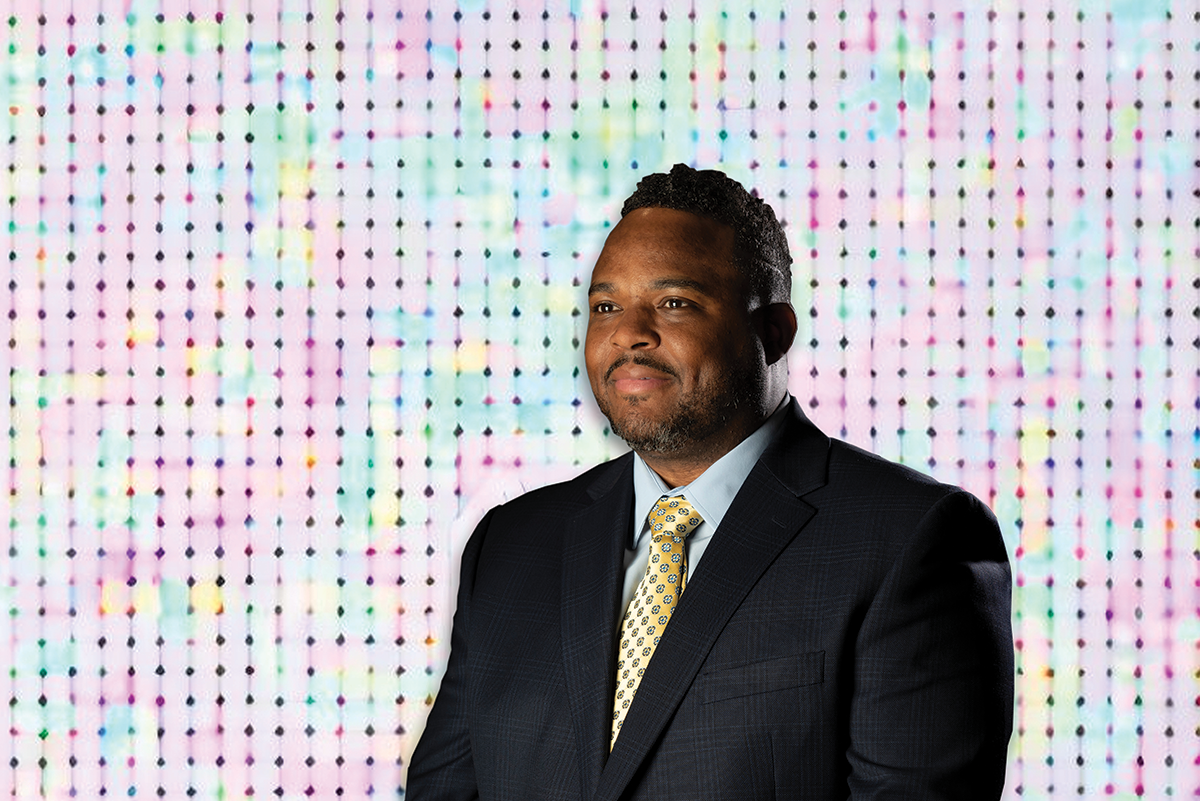
Photo by Les Todd. Background generated by AI.
One of the first requirements for improving equity in risk assessment is that the design team of an algorithm should be diverse in racial and ethnic background, as well as in profession, Cary said. “We think that math is unbiased,” he said. “I wouldn’t argue that it isn’t, but the producers of the math can be biased, because we’re talking about human beings.” Lack of diversity in racial or ethnic background among members of a design team increases the risk of introducing unconscious bias in algorithm design, he said.
Surprisingly, Cary, Pencina, and colleagues found that many algorithms don’t include health care providers — the end user — as part of the design team. “There is relatively little professional diversity in the design of these algorithms outside of data scientists,” Cary said. He, Pencina, and colleagues conducted the largest review of bias mitigation strategies used in health care algorithms to date and found that in over 100 published studies, even when health care professionals were involved on project teams, they were almost always physicians. That leaves out other practitioners such as therapists and nurses, who often deliver the bulk of bedside care to patients. “That is a design flaw,” he said. The review was published in October 2023 in the journal Health Affairs.
The Importance of Guardrails
Cary is developing research priorities and best practices for mitigating bias in use of AI in health care. For instance, he and collaborators are developing algorithms using a “health equity by design” approach, aiming to predict the risk of hospitalization.
"The overarching goal is not solely to decrease rehospitalization rates among all patients, but to achieve this in an equitable manner that minimizes disparities and variations between different subgroups. Right now, there are no standard guidelines for clinicians and algorithm developers to follow, so we’re trying to shed some light."
-Michael Cary, PhD, RN
He also serves on an oversight committee that reviews all clinical algorithms in use at Duke Health to ensure they meet quality standards, have been designed with equity in mind, and will improve patient care. The committee is part of the Algorithm-Based Clinical Decision Support (ABCDS) Oversight program, a collaboration between the School of Medicine and the Duke University Health System to ensure that all clinical algorithms in use are registered and reviewed.
In developing the program, Pencina, along with Nicoleta Economou-Zavlanos, PhD, and colleagues created a process akin to the one employed by the Food and Drug Administration for approval of medical devices, incorporating key best practices from software development. Developers register the algorithm, then submit project aims, along with evidence that the technology shows benefit, safety, and fairness, and future plans for deployment and funding.
“The significance of this lies in our careful governance and review process for algorithmic technologies throughout their development and utilization lifecycle,” said Economou-Zavlanos, director of ABCDS Oversight. “Through the creation of an algorithm inventory, we have gained a transparent system of accountability, enabling us to identify the individuals responsible, which is crucial in the event of any mishaps.” Duke University Health System has 54 registered tools or algorithms in use, about 39 of which use AI, she said. In addition to evaluating algorithms, the oversight program also educates the Duke community about health AI best practices.
Duke led the nation in putting this oversight into place early in 2021, ahead of the Food and Drug Administration’s national guidelines for health care algorithms issued in 2022. Now the Duke team is providing guidance at a national level as part of The Coalition for Health AI (CHAI), a community of academic health systems, industry partners, and AI practitioners that have come together to provide national guidelines.
Such “guardrails” are vital to realizing the promise of using AI in health care, Pencina said. He encourages developers to point AI to well-defined tasks, define the expected outcomes, and design the tool with the end user in mind. “We have a huge potential to reduce physician burden, increase health care efficiency, and improve the patient experience,” he said. “But we need to be very intentional about what AI will be doing.”
Story originally published in DukeMed Alumni News, Fall 2023.
Read more from DukeMed Alumni News
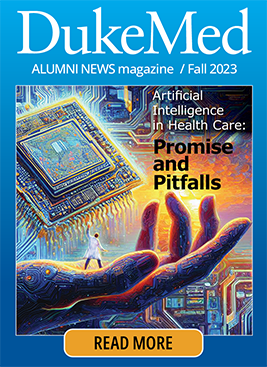